El Departamento de Defensa de EE UU ha redoblado sus esfuerzos en favor de un ejército basado en datos y potenciado por la inteligencia artificial. Sin embargo, el uso de la IA para aplicaciones de misión crítica para la defensa nacional se ha visto obstaculizado por una razón en particular: las alucinaciones. Las alucinaciones ocurren cuando los grandes modelos de lenguaje (LLM, por sus siglas en inglés) como ChatGPT generan información que suena plausible pero que es incorrecta en los hechos. No es raro que los LLM alucinen con una frecuencia de hasta 1 de cada 10 respuestas, según un estudio realizado por investigadores de la Universidad Carnegie Mellon. Es esa tasa de error del 10 por ciento la que ha frenado el potencial de la IA en el Departamento de Defensa.
The Defense Department has doubled down on a data-driven military empowered by artificial intelligence. The use of AI for mission-critical applications for national defense, however, has been hindered by one reason in particular – hallucinations.
Hallucinations happen when large language models (LLMs) such as ChatGPT generate plausible-sounding but factually incorrect information. It’s not uncommon for LLMs to hallucinate as often as 1 in every 10 responses, according to a study by researchers at Carnegie Mellon University. It’s that 10-percent error rate that’s slowed a fuller potential for AI in the DoD.
Now, however, there’s a new software solution called Retrieval Augmented Generation Verification (RAG-V) that addresses hallucinations in LLMs by drastically reducing their occurrence. Introduced by Primer, which builds practical and trusted AI for complex enterprise environments, RAG-V nearly eliminates hallucinations by adding a novel verification stage.
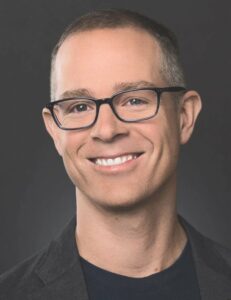
“RAG-V makes it possible to take a Large Language Model and put it into a mission-critical setting so warfighters can rely on it; that’s the heart of the matter,” said John Bohannon, vice president for data science at Primer. “In some settings you want hallucinations; it’s called creativity, such as when you want ideas for throwing a party. These models are very creative and come up with stuff out of thin air.
“The dark side is if you’re using it in a setting where factuality matters in getting answers to questions, hallucinations can be bad – especially when they’re subtle. When it’s an obviously incorrect piece of information, it’s easy for a human to catch it. The dangerous thing is when the model’s confident and it looks correct, but it’s a hallucination saying it in such a plausible, credible way that it can fool you.”
Primer works by incorporating a verification step akin to fact checking that grounds the LLM’s responses in substantiated data sources, reducing the above-mentioned error rate from around 5-10 percent – which is the state-of-the-art for leading LLMs – to just 0.1 percent. This dramatic improvement in reliability is critical for applications where factual accuracy is paramount, enabling warfighters and analysts to trust the AI-generated insights and make time-sensitive decisions with increased confidence.
Beyond detecting hallucinations, RAG-V also provides detailed explanations of any errors, allowing the system to iteratively improve and further enhance trust. Primer’s approach represents a significant advancement in responsible AI development, addressing key challenges around transparency and accountability that are essential for the adoption of these transformative technologies in the defense sector.
RAG-V reduces hallucinations
High-profile AI hallucinations have already made the news, especially in the legal profession where a lawyer submitted a court brief partially created with ChatGPT that invented case law.
It’s not a mystery why hallucinations happen, though. They’re caused by the way LLMs are trained. Even though they seem to be a mysterious piece of new science, they’re trained in a relatively simple manner that’s like a game of fill-in-the-blank. The LLM is fed text, for example, with some of the words hidden and the model fills in the blanks.
Do that billions of times and the LLM becomes very good at putting words together – just not always the right words. What the model’s trying to do is generate words that seem as high probability as possible, and read like they would’ve been written by a human.
A partial solution for hallucinations came on the scene in 2021 called Retrieval Augmented Generation – without the verification part created by Primer – that mitigated hallucinations down to the level they’re at today. RAG works by retrieving relevant information from a trusted system of record and then including it in the prompt for the generative model. The prompt also instructs the model to answer the user’s question only on that retrieved data without filling in the blanks.
Even with RAG, these models don’t always follow direction and still make errors and hallucinate. What Primer has done to bring down hallucinations to the point where trust can be restored on the part of the user is add a new, last step that fact checks the data against verifiable sources – hence the verification in RAG-V.
With RAG-V, warfighters executing mission-critical applications can now add a trustworthy LLM capability to their toolkit in these times of great competitors also developing advanced AI capabilities where operations across multiple domains and at the edge are needed.
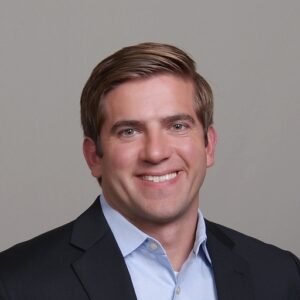
“In order for us to stay ahead of competitors like China, Russia, Iran, and North Korea – all of whom are using LLMs – our AI at its core is about helping the end users do something more efficiently by doing it with less effort in a faster manner,” said Matthew Macnak, senior vice president of customer solutions engineering at Primer. “Primer is a full platform; it’s not just powered by an LLM so we can actually take a version of that into the field and still have the ability to process massive amounts of unstructured information with fewer personnel and more reliability due to RAG-V.
“Imagine that we can put our AI systems into something the size of a suitcase and then place it on an aircraft that’s doing ISR missions. Now you have a few operators able to ingest, let’s say, thousands of voice or text collects and analyze that information in real time rather than just collecting the data, going back, and waiting for the data to be analyzed. Now they can have a result in situ rather than waiting on someone else to tell them what to do.”
More trust, fewer hallucinations
Even with RAG, Large Language Models have error rates so bad – typically 5-10 percent of the time – that they can’t be trusted for many defense applications, as highlighted. The rate depends on the data and what questions are being asked of the LLM, but that makes them unacceptable for intelligence operations, for example.
“If you have something that’s going to fib one out of every 10 times you ask it a question, that means that you have to check its work every single time,” noted Bohannon. “You have to be hypervigilant and therefore that’s a deal breaker in a setting where you’re using this to actually augment a human. When the stakes are high, you can’t afford to have to use a tool that you can’t trust.”
When it comes to LLMs, Bohannon suggests being a skeptical buyer. When an analyst’s need to brainstorm can be addressed with ChatGPT, for example, they’re likely in safe territory regarding hallucinations. But if the need is mission critical with high stakes, users should be wary of LLMs without a final step for verification and fact checking.
“Even when they’re used with best practices like RAG and other guardrails for Large Language Models, they still have an unacceptable error rate. Potential users should come in with the mindset that this technology is new and there’s only a few shops out there like Primer that are taking that problem seriously.”
Added Macnak: “We’ve all heard ‘trust but verify.’ The valuable part of RAG-V is that it allows the user to see exactly why it made a decision and more importantly how that output was verified. Our focus remains on the end user, whether that’s an analyst, operator, or warfighter.
“To that end, we’re building products and technology around those individuals that actually work in order to serve them. The 0.1 percent error rate we’ve reached with RAG-V is a great example of why we’re doing this for users making mission-critical decisions.”
Fuente: https://breakingdefense.com