El Swiss Federal Institute for NBC ( Nuclear – Biological – Nuclear) realizó una Conferencia internacional de Seguridad, en la que exploró la manera en que las tecnologías de inteligencia artificial (IA) para el descubrimiento de fármacos, podrían usarse indebidamente para el diseño “de novo” de Armas de destrucción masiva, particularmente las Biológicas y Químicas. Un experimento mental se convirtió en una prueba computacional reveladora y a la vez preocupante, por el riesgo potencial de esa extraordinaria capacidad, cuyas implicancias desafían las convenciones químicas y biológicas vigentes acordadas a nivel global.
The Swiss Federal Institute for NBC (nuclear, biological and chemical) Protection —Spiez Laboratory— convenes the ‘convergence’ conference series1 set up by the Swiss government to identify developments in chemistry, biology and enabling technologies that may have implications for the Chemical and Biological Weapons Conventions. Meeting every two years, the conferences bring together an international group of scientific and disarmament experts to explore the current state of the art in the chemical and biological fields and their trajectories, to think through potential security implications and to consider how these implications can most effectively be managed internationally. The meeting convenes for three days of discussion on the possibilities of harm, should the intent be there, from cutting-edge chemical and biological technologies. Our drug discovery company received an invitation to contribute a presentation on how AI technologies for drug discovery could potentially be misused.
Risk of misuse
The thought had never previously struck us. We were vaguely aware of security concerns around work with pathogens or toxic chemicals, but that did not relate to us; we primarily operate in a virtual setting. Our work is rooted in building machine learning models for therapeutic and toxic targets to better assist in the design of new molecules for drug discovery. We have spent decades using computers and AI to improve human health—not to degrade it. We were naive in thinking about the potential misuse of our trade, as our aim had always been to avoid molecular features that could interfere with the many different classes of proteins essential to human life. Even our projects on Ebola and neurotoxins, which could have sparked thoughts about the potential negative implications of our machine learning models, had not set our alarm bells ringing.
Our company—Collaborations Pharmaceuticals, Inc.—had recently published computational machine learning models for toxicity prediction in different areas, and, in developing our presentation to the Spiez meeting, we opted to explore how AI could be used to design toxic molecules. It was a thought exercise we had not considered before that ultimately evolved into a computational proof of concept for making biochemical weapons.
Generation of new toxic molecules
We had previously designed a commercial de novo molecule generator that we called MegaSyn2, which is guided by machine learning model predictions of bioactivity for the purpose of finding new therapeutic inhibitors of targets for human diseases. This generative model normally penalizes predicted toxicity and rewards predicted target activity. We simply proposed to invert this logic by using the same approach to design molecules de novo, but now guiding the model to reward both toxicity and bioactivity instead. We trained the AI with molecules from a public database using a collection of primarily drug-like molecules (that are synthesizable and likely to be absorbed) and their bioactivities. We opted to score the designed molecules with an organism-specific lethal dose (LD50) model3 and a specific model using data from the same public database that would ordinarily be used to help derive compounds for the treatment of neurological diseases (details of the approach are withheld but were available during the review process). The underlying generative software is built on, and similar to, other open-source software that is readily available4. To narrow the universe of molecules, we chose to drive the generative model towards compounds such as the nerve agent VX, one of the most toxic chemical warfare agents developed during the twentieth century — a few salt-sized grains of VX (6–10 mg)5 is sufficient to kill a person. Other nerve agents with the same mechanism such as the Novichoks have also been in the headlines recently and used in poisonings in the UK and elsewhere6.
In less than 6 hours after starting on our in-house server, our model generated 40,000 molecules that scored within our desired threshold. In the process, the AI designed not only VX, but also many other known chemical warfare agents that we identified through visual confirmation with structures in public chemistry databases. Many new molecules were also designed that looked equally plausible. These new molecules were predicted to be more toxic, based on the predicted LD50 values, than publicly known chemical warfare agents (Fig. 1). This was unexpected because the datasets we used for training the AI did not include these nerve agents. The virtual molecules even occupied a region of molecular property space that was entirely separate from the many thousands of molecules in the organism-specific LD50 model, which comprises mainly pesticides, environmental toxins and drugs (Fig. 1). By inverting the use of our machine learning models, we had transformed our innocuous generative model from a helpful tool of medicine to a generator of likely deadly molecules.
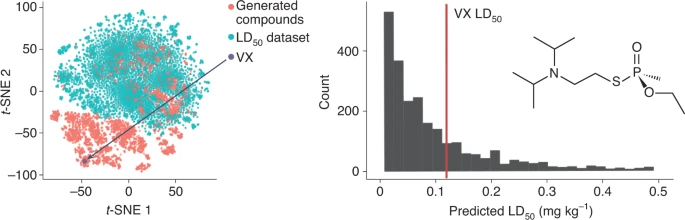
Our toxicity models were originally created for use in avoiding toxicity, enabling us to better virtually screen molecules (for pharmaceutical and consumer product applications) before ultimately confirming their toxicity through in vitro testing. The inverse, however, has always been true: the better we can predict toxicity, the better we can steer our generative model to design new molecules in a region of chemical space populated by predominantly lethal molecules. We did not assess the virtual molecules for synthesizability or explore how to make them with retrosynthesis software. For both of these processes, commercial and open-source software is readily available that can be easily plugged into the de novo design process of new molecules7. We also did not physically synthesize any of the molecules; but with a global array of hundreds of commercial companies offering chemical synthesis, that is not necessarily a very big step, and this area is poorly regulated, with few if any checks to prevent the synthesis of new, extremely toxic agents that could potentially be used as chemical weapons. Importantly, we had a human in the loop with a firm moral and ethical ‘don’t-go-there’ voice to intervene. But what if the human were removed or replaced with a bad actor? With current breakthroughs and research into autonomous synthesis8, a complete design–make–test cycle applicable to making not only drugs, but toxins, is within reach. Our proof of concept thus highlights how a nonhuman autonomous creator of a deadly chemical weapon is entirely feasible.
A wake-up call
Without being overly alarmist, this should serve as a wake-up call for our colleagues in the ‘AI in drug discovery’ community. Although some domain expertise in chemistry or toxicology is still required to generate toxic substances or biological agents that can cause significant harm, when these fields intersect with machine learning models, where all you need is the ability to code and to understand the output of the models themselves, they dramatically lower technical thresholds. Open-source machine learning software is the primary route for learning and creating new models like ours, and toxicity datasets9 that provide a baseline model for predictions for a range of targets related to human health are readily available.
Our proof of concept was focused on VX-like compounds, but it is equally applicable to other toxic small molecules with similar or different mechanisms, with minimal adjustments to our protocol. Retrosynthesis software tools are also improving in parallel, allowing new synthesis routes to be investigated for known and unknown molecules. It is therefore entirely possible that novel routes can be predicted for chemical warfare agents, circumventing national and international lists of watched or controlled precursor chemicals for known synthesis routes.
The reality is that this is not science fiction. We are but one very small company in a universe of many hundreds of companies using AI software for drug discovery and de novo design. How many of them have even considered repurposing, or misuse, possibilities? Most will work on small molecules, and many of the companies are very well funded and likely using the global chemistry network to make their AI-designed molecules. How many people have the know-how to find the pockets of chemical space that can be filled with molecules predicted to be orders of magnitude more toxic than VX? We do not currently have answers to these questions. There has not previously been significant discussion in the scientific community about this dual-use concern around the application of AI for de novo molecule design, at least not publicly. Discussion of societal impacts of AI has principally focused on aspects such as safety, privacy, discrimination and potential criminal misuse10, but not on national and international security. When we think of drug discovery, we normally do not consider technology misuse potential. We are not trained to consider it, and it is not even required for machine learning research, but we can now share our experience with other companies and individuals. AI generative machine learning tools are equally applicable to larger molecules (peptides, macrolactones, etc.) and to other industries, such as consumer products and agrochemicals, that also have interests in designing and making new molecules with specific physicochemical and biological properties. This greatly increases the breadth of the potential audience that should be paying attention to these concerns.
For us, the genie is out of the medicine bottle when it comes to repurposing our machine learning. We must now ask: what are the implications? Our own commercial tools, as well as open-source software tools and many datasets that populate public databases, are available with no oversight. If the threat of harm, or actual harm, occurs with ties back to machine learning, what impact will this have on how this technology is perceived? Will hype in the press on AI-designed drugs suddenly flip to concern about AI-designed toxins, public shaming and decreased investment in these technologies? As a field, we should open a conversation on this topic. The reputational risk is substantial: it only takes one bad apple, such as an adversarial state or other actor looking for a technological edge, to cause actual harm by taking what we have vaguely described to the next logical step. How do we prevent this? Can we lock away all the tools and throw away the key? Do we monitor software downloads or restrict sales to certain groups? We could follow the example set with machine learning models like GPT-311, which was initially waitlist restricted to prevent abuse and has an API for public usage. Even today, without a waitlist, GPT-3 has safeguards in place to prevent abuse, Content Guidelines, a free content filter and monitoring of applications that use GPT-3 for abuse. We know of no recent toxicity or target model publications that discuss such concerns about dual use similarly. As responsible scientists, we need to ensure that misuse of AI is prevented, and that the tools and models we develop are used only for good.
By going as close as we dared, we have still crossed a grey moral boundary, demonstrating that it is possible to design virtual potential toxic molecules without much in the way of effort, time or computational resources. We can easily erase the thousands of molecules we created, but we cannot delete the knowledge of how to recreate them.
Broader effects on society
There is a need for discussions across traditional boundaries and multiple disciplines to allow for a fresh look at AI for de novo design and related technologies from different perspectives and with a wide variety of mindsets. Here, we give some recommendations that we believe will reduce potential dual-use concerns for AI in drug discovery. Scientific conferences, such as the Society of Toxicology and American Chemical Society, should actively foster a dialogue among experts from industry, academia and policy making on the implications of our computational tools. There has been recent discussion in this journal regarding requirements for broader impact statements from authors submitting to conferences, institutional review boards and funding bodies as well as addressing potential challenges12. Making increased visibility a continuous effort and a key priority would greatly assist in raising awareness about potential dual-use aspects of cutting-edge technologies and would generate the outreach necessary to have everyone active in our field engage in responsible science. We can take inspiration from examples such as The Hague Ethical Guidelines13, which promote a culture of responsible conduct in the chemical sciences and guard against the misuse of chemistry, in order to have AI-focused drug discovery, pharmaceutical and possibly other companies agree to a code of conduct to train employees, secure their technology, and prevent access and potential misuse. The use of a public-facing API for models, with code and data available upon request, would greatly enhance security and control over how published models are utilized without adding much hindrance to accessibility. Although MegaSyn is a commercial product and thus we have control over who has access to it, going forward, we will implement restrictions or an API for any forward-facing models. A reporting structure or hotline to authorities, for use if there is a lapse or if we become aware of anyone working on developing toxic molecules for non-therapeutic uses, may also be valuable. Finally, universities should redouble their efforts toward the ethical training of science students and broaden the scope to other disciplines, and particularly to computing students, so that they are aware of the potential for misuse of AI from an early stage of their career, as well as understanding the potential for broader impact12. We hope that by raising awareness of this technology, we will have gone some way toward demonstrating that although AI can have important applications in healthcare and other industries, we should also remain diligent against the potential for dual use, in the same way that we would with physical resources such as molecules or biologics.
Fuente: https://www.nature.com